Introduction
The diffusion of artificial intelligence (AI) enabled systems and technologies has sparked new debates on whether AI can fundamentally reshape strategic, operational, and tactical decision-making in warfare. James Johnson (2022a), for example, argues that AI capabilities “cannot effectively or reliably compliment (let alone replace) the role of humans in understanding and apprehending the strategic environment, or make predictions and judgements that inform strategic decisions.” Boulanin et. al. (2020) also provide a comprehensive overview and critique as to why using AI-enabled capabilities cannot reliably and safely augment command and control (C2) decision-making processes. The prevailing argument is that the ‘fog and friction’ of warfare – the uncertainty, complexity, and unpredictability of warfare – cannot be subjected to inductive machine learning logic. Furthermore, the growing range of ethical, moral, and legal challenges of applying AI in military decision-making and operations stipulates even greater strategic importance in preserving human control in AI-enabled warfare (Payne, 2021, Johnson 2021).
Notwithstanding the ongoing validity of these works and arguments, this article attempts to provide a contending perspective – arguing that the diffusion of AI can enhance the pace, accuracy, and adaptability of military operations, particularly in air power, in ways previously unimaginable. In particular, traditional OODA loop concepts, developed by John Boyd (1987), have relied on the idea of exploiting situational awareness with rapid analysis to gain an advantage over adversaries – in other words, optimizing human decision-making in high-tempo combat operations, while serving as an interface between a human (pilot) and machine (aircraft). In the age of AI, however, AI’s capabilities may allow air forces to move from sequential, human-centered decision cycles to high-speed, automated OODA loops that could allow for responses in near-real time. This shift is essential as modern air combat and air defense require processing massive quantities of information from disparate and distributed sources, analyzing complex patterns, and reacting to threats with unprecedented speed—all areas where various AI systems aim to excel.
Arguably, the diffusion and advancement of AI technologies change the traditional OODA loop concept (Boyd, 1987) in two significant ways: first, by transforming data processing and analysis in the Observe and Orient phases, enabling faster, more accurate insights than human operators can manage, and second, by integrating predictive and autonomous decision-making capabilities, which compress the Decide and Act phases into almost seamless action. AI, for example, can sift through and interpret vast amounts of sensor and operational data, detect patterns, predict adversary movements, and trigger appropriate responses within milliseconds. This acceleration of the OODA loop not only outpaces human cognition but also restricts the window for time-critical responses to adversaries, creating a potential advantage in high-tempo combat operations. By embedding AI throughout the OODA process, future air force doctrine can establish a highly adaptive capability to respond to changes in the operational environment – creating “Super OODA” loops that may effectively neutralize or mitigate adversarial actions even before they occur. Thus, AI systems do not simply speed up the OODA loop; they fundamentally redefine the traditional operational cycle as a more fluid and seamless process that leverages automation to stay perpetually ahead of evolving threats and adversarial decision-making.
For air forces, increasing the effectiveness of decision cycles this way—termed by the author as creating “Super OODA Loops”—offers the potential to achieve force superiority in fast-paced, high-intensity operational environments. The term “Super OODA Loop” can be conceptualized at two major aspects: On one hand, as an optimized, accelerated OODA process that leverages advanced technologies, including AI, machine learning, and data integration to enhance situational awareness, orientation, and decision-making speed to outpace adversaries. On the other hand, Super OODA Loop can be also viewed as an advanced operational framework that integrates the varying OODA loops of allied air forces, enabling collaboration and information-sharing across different military units and branches. This integration aims to enhance shared situational awareness, where each entity contributes its unique insights and data to create a comprehensive common operational picture. By synchronizing the Observe, Orient, Decide, and Act phases across allied forces, the Super OODA Loop could facilitate faster and more informed decision-making, maximizing military effectiveness and adaptability in dynamic operational scenarios.
AI systems do not simply speed up the OODA loop; they fundamentally redefine the traditional operational cycle as a more fluid and seamless process that leverages automation to stay perpetually ahead of evolving threats and adversarial decision-making.
Dr. Michael Raska
Theoretical Foundations of Super OODA Loops: Past, Present, and Future Trajectories
The evolution of the OODA loop from its origins to a “Super OODA Loop” reflects a possible conceptual iteration of harnessing cutting-edge technology to enhance decision-making cycles by air forces. The OODA loop’s purpose remains the same: to establish decision superiority over adversaries by observing, orienting, deciding, and acting faster than the opponent (Boyd, 1987). However, the tools and methodologies that support each phase have dramatically changed over time, driven by novel advances in information technology, sensor systems, AI, and data fusion. By examining past, present, and future trajectories, summarized in Table 2.1 below, it is possible to build an understanding of how Super OODA Loops can change traditional conceptual approaches to create a faster, more autonomous, and more predictive decision-making process (Osinga, 2013, Johnson 2022a).
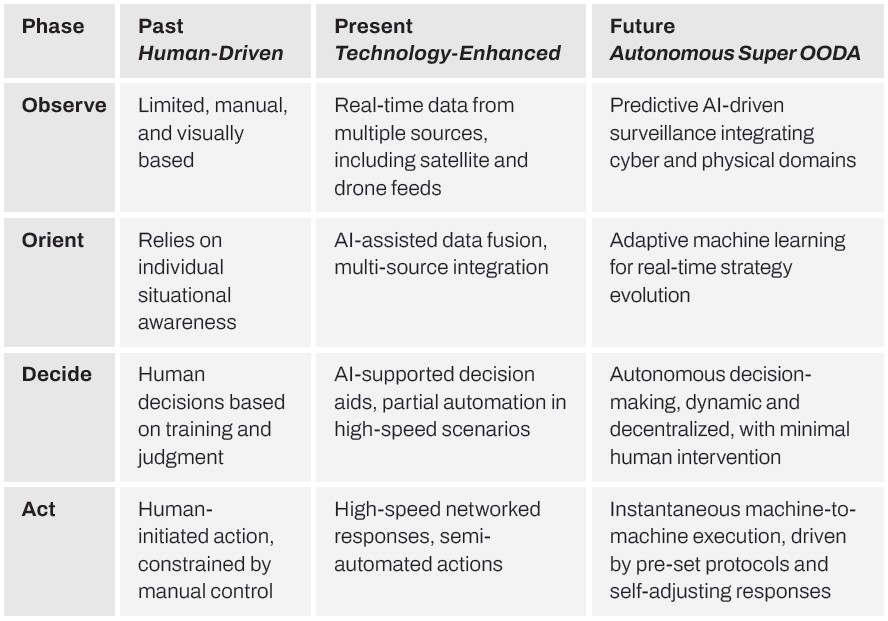
The Past: Human-Driven OODA Loops
In the past, OODA loops were primarily manual, human-driven processes that relied on direct observation, limited inputs from sensors, and tactical radio communication. For example, in air combat during the Korean and Vietnam wars, pilots gathered intelligence directly from visual cues and basic radar systems. Orientation was heavily dependent on an individual pilot’s training and skill level, situational awareness, and real-time judgment. At the same time, decision-making and action relied on the ability to interpret environmental cues quickly and respond through reflexive manoeuvre. Information flowed through a hierarchical command structure, meaning that intelligence-gathering and operational or tactical decision-making were slowed by traditional command and control (C2) processes and relay communications, which were, in turn, limited by the information-processing capabilities of humans.
Early air doctrine emphasized training and experience to ensure that highly skilled pilots and commanders could adapt rapidly to unpredictable operational conditions. As a result, these human-driven OODA loops were effective at the time, even though they were constrained by the physiological limits of human cognition and reaction times. However, without real-time data integration, data processing, or predictive capabilities, these OODA loops were slower and more vulnerable to error and time-sensitive information requirements.
The Present: Technology-Enhanced OODA Loops
With advancements in digital technology, sensor integration, and AI, contemporary OODA loops in air power doctrines have evolved to become faster, more integrated, and partially automated. Present-day OODA loops are augmented by advanced sensing capabilities, such as multi-array radar, satellite imagery, electronic warfare (EW) systems, and more dynamic C2 and battle management networks (Work & Brimley, 2014). These technologies enhance each phase of the OODA process:
- Observe: Modern OODA loops gather data from a range of networked sensors, including surveillance drones, satellites, air- and ground-based radar systems. Information flows in real-time, providing a detailed and continuously updated multi-domain picture of the battlespace.
- Orient: AI and machine learning algorithms play a significant role in synthesizing and interpreting the tremendous influx of data and information, allowing operators to quickly gain situational awareness by helping identify patterns, filter relevant information, and flag potential threats. Orientation is further supported by data fusion to integrate intelligence from various sources into a common operational picture.
- Decide: AI-assisted decision-support systems analyze evolving changes in the operating environment and predict potential outcomes, suggesting optimal courses of action to human operators (Cummings, 2021). This allows for faster decision-making in complex environments where split-second choices can make the difference between success and failure. In some cases, these systems can make autonomous decisions for specific high-speed applications, such as missile defense and other automated threat responses.
- Act: High-speed networks allow C2 and battle management to be executed rapidly, while automated systems can be programmed to act on certain conditions with minimal human oversight. For instance, in defense operations, systems can autonomously deploy electronic countermeasures (ECM) against incoming threats, compressing the Decide and Act phases into one seamless action.
Today’s technology-enhanced OODA loops already create a “Super OODA” compared to the original human-centric decision-making design. However, even these advanced loops still rely heavily on human oversight, especially in high-stakes decision-making involving lethal action. While faster and more comprehensive, today’s OODA loops continue to be limited by latency in the information-processing communication networks, human decision-making bottlenecks, and various constraints relating to AI autonomy.
The Future: Autonomous, Predictive, and Allied ‘Super OODA Loops’
Looking ahead, the trajectory of OODA loops points toward increasing levels of automation, interconnectivity, and the use of predictive analytics in air power. The future Super OODA Loop will be increasingly AI-driven, more autonomous, and capable of operating in near-instantaneous timelines that massively outpace human cognition and response times (Scharre, 2023). Three main developments can be anticipated in the direction and character of future Super OODA Loops:
- Real-Time Data Integration and Predictive Observations: Next-generation OODA loops will gather data from a wide array of sources, including AI-enhanced surveillance systems and cyber intelligence feeds, with edge computing allowing for the rapid processing of vast volumes of information remotely. AI algorithms will detect complex patterns in data to predict or see adversarial actions before they occur, transforming the Observe phase into a pre-emptive, rather than purely reactive, step (Johnson, 2022).
- Adaptive Orientation Through Machine Learning: Future Super OODA Loops will continuously refine warfighter orientation through machine learning. These algorithms will support warfighters in adapting to opponent tactics quickly by learning from each encounter and exploiting historical datasets to improve decision quality. Machine learning will also allow for more complex data synthesis and pattern recognition, potentially identifying subtle cues that indicate shifts in the adversary’s planning and decision-making, enhancing the OODA loop’s ability to orient itself more dynamically.
- Autonomous Decision-Making and Immediate Action: With improved AI models and ethical frameworks, specific decision-making processes will become fully autonomous. These systems may act independently in scenarios where milliseconds are critical, such as threat interception or defending against advanced drone swarms. In the Act phase, C2 will bypass traditional human-on-loop approval, instead relying on pre-defined rules of engagement, protocols, and machine-to-machine communication to initiate action at machine speed. This will create a rapid decision-action loop where the AI autonomously adjusts response based on real-time feedback from the environment.
As emerging technologies reach maturity, Super OODA Loops will become less reliant on human intervention, particularly in environments where decision-action cycles need to be faster than human cognition can manage (Horowitz, 2022). For example, future air power doctrine will incorporate squadrons of uncrewed combat autonomous vehicles (UCAVs) and other AI-enabled, self-directing platforms that can operate remotely across distributed battle networks, make collaborative decisions with human and machine partners, and maneuver faster than adversaries can detect, orient, or counter (Layton, 2023). Figure 2.2 illustrates how various emerging technologies can augment the traditional OODA loop.
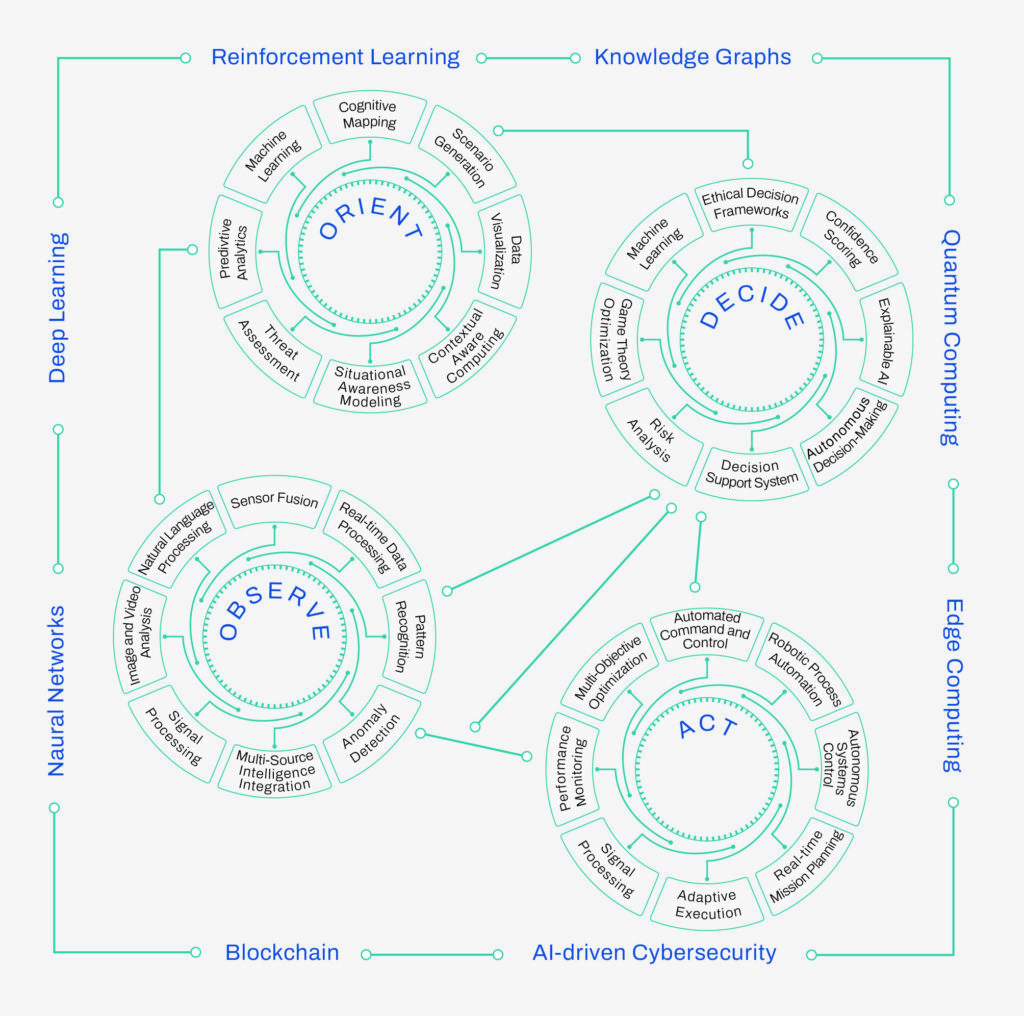
Operational Implementation in Air Force Doctrines
The future Super OODA Loop concept represents a fundamental shift from human-aided automation to highly autonomous, machine-driven decision cycles. This evolution elevates existing as well as new challenges—such as ensuring robust cybersecurity, developing highly interoperable and adaptable force structures, and defining the ethical boundaries for autonomous lethal action—but also promises a level of operational speed and flexibility unmatched by legacy approaches. Future trajectories point to air combat doctrines being redefined for a new technological environment and will demand air forces evolve operational constructs and training to harness the full potential of the Super OODA Loop. Implementing Super OODA Loops in air force doctrine will require both technology integration and doctrinal shift across a wide spectrum of mission profiles. The Super OODA Loop’s acceleration and partial fusion of the Observe, Orient, Decide, and Act phases could therefore enable faster and more effective responses in scenarios that demand rapid and dynamic responses.
Importantly, Super OODA Loops may also support the integration of situational awareness among allies and partners – allowing each allied force component to contribute its unique insights and intelligence, thereby fostering a more robust shared understanding of the operational environment. Additionally, the synchronization of decision-making processes across multiple entities would – in theory – facilitate accelerated decision-making with higher reliability and accuracy. By minimizing the time required for information analysis, allied forces would be able to respond more swiftly to emerging threats and dynamic operational scenarios, where timely action is vital for favourable outcomes. Consequently, the integrated nature of the Super OODA Loop could enhance the interoperability and adaptability among allied forces.
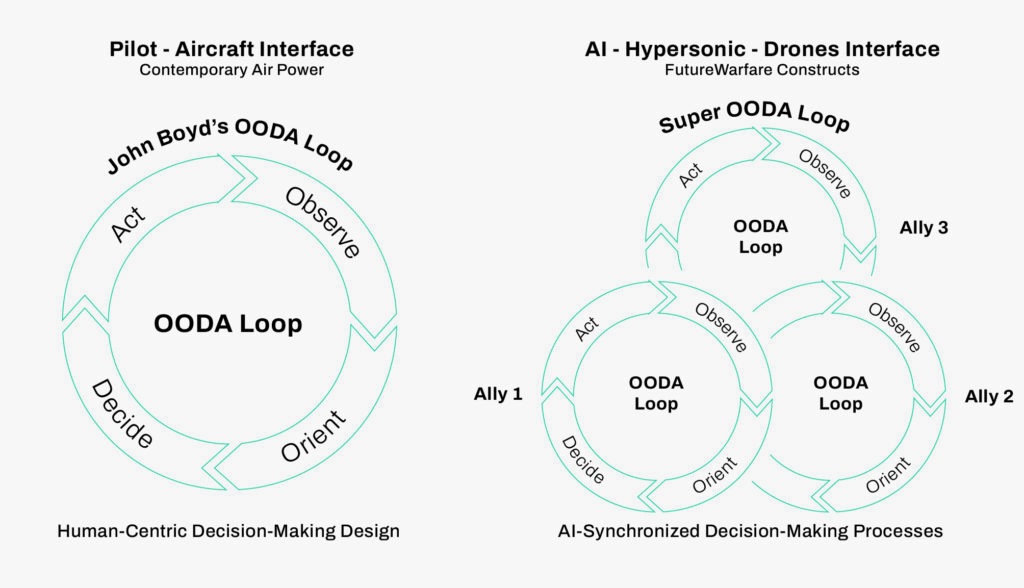
Air Superiority and Offensive Operations
In offensive operations, Super OODA Loops may support freedom of maneuver and prove critical for gaining air superiority by rapidly identifying, orienting to, and neutralizing threats ahead of adversarial decision-making and reaction timelines. Already, advanced real-time data analytics, such as the Distributed Common Ground System (DCGS) used by the U.S. Air Force, are combining intelligence from various sources—including airborne surveillance, signals intelligence, and space-based intelligence feeds—to create a holistic view of the battlespace (U.S. Air Force, 2024). DCGS processes data to identify hostile movements and vulnerabilities, accelerating the Observe and Orient phases, thereby allowing commanders to prioritize high-value targets and deploy resources more precisely where and when needed.
In a hypothetical air-to-ground strike scenario, AI-driven Super OODA Loops would streamline target identification and strike execution by autonomously processing multi-source intelligence to determine the precise geolocation of adversarial air defense assets and be able to recommend optimal flight paths and tasking orders for attack plans. Such an approach would optimize the kill chain by drastically shortening the time between target identification and engagement, minimizing the risk to operators and air assets by calculating the safest and most efficient flight paths, all within seconds. Advanced predictive analytics within these loops would also assess and predict potential adversarial courses of action and reactions based on past behaviors learned by the AI, allowing for pre-emptive countermeasures to prevent adversaries from responding effectively.
Training and Simulated Exercises
Training plays a crucial role in embedding Super OODA Loops into air power doctrine, as personnel must adapt to new decision-making dynamics. Simulated environments powered by AI and machine learning that replicate high-stakes, fast-paced combat scenarios provide an invaluable training ground for air crews and command officers to practice and exercise responses using new systems and processes (Reilly, 2023). The U.S. Air Force’s expanding use of virtual reality (VR) and AI-powered simulations creates a dynamic training environment where warfighters and operators exposed to dynamic operaional scenarios and advanced threat simulations can learn to react inside highly compressed timelines. By integrating AI-enabled scenarios that mimic the decision-making processes and tactics of potential adversaries, contemporary air combat exercises push pilots to continuously test and optimize OODA responses under realistic operating conditions. Additionally, training simulators equipped with machine learning can analyze and assess performance to pinpoint areas for improvement, offering personalized feedback that helps operators improve orientation and decision-making skills.
Challenges and Risks in Implementing Super OODA Loops
While Super OODA Loops offer compelling advantages, they also amplify challenges and risks that must be addressed for successful implementation. Key concerns converge on data overload, cybersecurity vulnerabilities, ethical considerations, and the integration of legacy systems.
Data Overload and Cognitive Saturation
The amount of data processed in real-time within a Super OODA Loop has the potential to overwhelm operators, risking cognitive saturation and analysis paralysis (Endsley, 2022). While beneficial, AI-based systems like the Distributed Common Ground System (DCGS) often produce vast amounts of information that require prioritization. For example, in scenarios with multiple high-priority threats, such as simultaneous enemy aircraft and missile engagements, operators may find it challenging to discern which threat demands the most immediate and time-sensitive action. This is compounded by the tendency for AI systems to provision insights at a speed that outpaces the ability of human operators to process them effectively.
To manage this, data-filtering algorithms must be harnessed to mitigate the risk of information burden, highlighting only the most critical data at any given moment during the mission cycle. Additionally, autonomous prioritization features could alleviate operator workload by selecting the specific threats that require urgent intervention versus those that can be managed through other pre-emptive or automated responses. The design of future Super OODA Loops will therefore demand advanced human systems integration and ensure application interfaces for operators safeguard against cognitive overload and allow operators to focus on high-level tasks and decision-making (Hoffman et al., 2024).
Cybersecurity and System Reliability
As Super OODA Loops rely heavily on AI and networked systems, they introduce new types of cybersecurity risks that adversaries will aim to exploit. Opponents could disrupt the OODA cycle by hacking communication networks, jamming sensors, or injecting false data (Arquilla & Ronfeldt, 2021). For example, adversarial cyber targeters could alter the data fed into AI-driven targeting systems, misleading forces into targeting false threats and locations. Countermeasure such as advanced encryption, network segmentation, and intrusion-detection systems, will be critical for securing the vast data flows across future OODA loops. Cybersecurity protocols will need to be optimized for a new risk framework to detect and counteract potential intrusions before they can impact operational security, resilience, and decision-making. Maintaining redundant systems that can operate independently, such as non-networked backup sensors, could ensure that even a compromised Super OODA Loop can function even in a degraded state.
Ethical and Legal Concerns
The autonomy within Super OODA Loops, especially in lethal contexts, raises significant ethical and legal issues. Autonomous decisions, such as engaging targets or executing pre-emptive kinetic effects without human oversight have the potential for unintended consequences, particularly in complex and benign operational environments. To address these concerns, it is essential to establish clear protocols and guidelines on where and when autonomous decisions are permissible in line with stringent rules of engagement. Human-in-the-loop or human-on-the-loop models—where human operators retain final control over critical decisions—will remain essential safeguards for such scenarios. For example, the U.S. Department of Defense’s ethical AI guidelines recommend that a human should remain involved in all lethal decision-making processes. These ethical guidelines must continue to evolve in tandem with technological advancements to ensure the responsible use of autonomous systems within Super OODA Loops.
Integration with Legacy Systems
Integrating Super OODA Loops into air power doctrine requires compatibility with existing platforms and infrastructure, many of which may not support real-time data processing or AI-enabled automation. For example, legacy fighter aircraft or radar systems that rely on older communication systems may not be able to fully leverage high-speed, interconnected networks that Super OODA Loops will run on. This can create interoperability barriers and reduce the overall effectiveness of the OODA loop. Retrofitting legacy platforms with modular components to enhance connectivity and data-processing capabilities, where possible, will be necessary. The U.S. Air Force’s Open Mission Systems (OMS) initiative exemplifies this approach by creating modular, open-architecture systems that allow older assets to interface with newer, AI-assisted platforms. With a phased adoption strategy where modular upgrades can be implemented, air forces can transition legacy systems into a Super OODA environment without overhauling entire fleets or infrastructures.
Conclusion
Implementing Super OODA Loops within air power doctrines amplifies the ambitious promise of providing significant changes and advantages in terms of speed, adaptability, and operational precision in future air power. By implementing the Super OODA Loops, reflected in this paper, may improve air force capabilities across a wide range of missions, from air superiority to warfighter training, allowing faster detection, orientation, decision-making, and action. However, fully realizing the potential of Super OODA Loops requires addressing complex challenges, including data overload, cybersecurity, ethical considerations, and interoperability with legacy systems. By navigating these issues through strategic and policy debates, professional military education and training, and making doctrines more adaptive to accelerating technological breakthroughs, air forces can harness the power of Super OODA Loops to achieve decision superiority and, in doing so, maintain a competitive edge in the future battlespace.
References
Arquilla, J., and Ronfeldt, D. (2021). Cyberwar is coming! In Cyberwar is Coming!. RAND Corporation, pp. 23-60. Available from: https://doi.org/10.1234/rand.cyberwar.2021
Boyd, J. R. (1987) A discourse on winning and losing. Maxwell Air Force Base, AL: Air University Library Document No. M-U 43947. Available from: https://www.airuniversity.af.edu/AUPress/Display/Article/1534556/
Boulanin, Vincent (ed.) (2020). Artificial Intelligence, Strategic Stability and Nuclear Risk. Stockholm: SIPRI Publications. Available from: https://www.sipri.org/publications/2020/policy-reports/artificial-intelligence-strategic-stability-and-nuclear-risk
Cummings, M. L. (2021) Artificial intelligence and the future of warfare. Chatham House. Available from: https://www.chathamhouse.org/2021/06/artificial-intelligence-and-future-warfare
Endsley, M. R. (2022) Situation awareness in future autonomous air and space systems. Journal of Cognitive Engineering and Decision Making, 16(1), 23-47. Available from: https://doi.org/10.1177/15553434221234567
Hoffman, R. R., Klein, G., & Mueller, S. T. (2024) The evolution of cognitive systems engineering. IEEE Intelligent Systems, 39(1), 7-18. Available from: https://doi.org/10.1109/MIS.2024.1234567
Horowitz, M. C. (2022) Artificial intelligence, international competition, and the balance of power. Texas National Security Review, 5(2), 10-33. Available from: https://doi.org/10.15781/T2AB1234
Johnson, J. (2021). Artificial Intelligence & the Future of Warfare: USA, China, and Strategic Stability. Manchester: Manchester University Press
Johnson, J. (2022a). Automating the OODA loop in the age of intelligent machines: reaffirming the role of humans in command-and-control decision-making in the digital age. Defence Studies, 23(1), 43–67. Available from: https://doi.org/10.1080/14702436.2022.2102486
Johnson, J. (2022b). Artificial intelligence and future warfare: Implications for international security. Defense & Security Analysis, 38(1), 36–54. Available from: https://doi.org/10.1080/14751798.2022.1234567
Layton, P. (2023) Algorithmic warfare: AI-enabled combat aircraft in future air operations. Air & Space Power Journal, 37(2), 4–20. Available from: https://www.airuniversity.af.edu/ASPJ/Article/1234567/
Osinga, F. (2013) Getting’ A Discourse on Winning and Losing: A Primer on Boyd’s ‘Theory of Intellectual Evolution’. Contemporary Security Policy 34(3), 603–624. Available from: https://doi.org/10.1080/13523260.2013.849154
Payne, K. (2021). Warbot: The Dawn of Artificially Intelligent Conflict. New York: Oxford University Press.
Reilly, M. B. (2023) Virtual reality and AI in military training: Enhancing decision-making in complex environments. Military Review, 103(4), 68–79. Available from: https://www.armyupress.army.mil/Journals/Military-Review/1234567/
Scharre, P. (2023) Autonomous weapons and operational risk. Center for a New American Security. Available from: https://www.cnas.org/publications/reports/autonomous-weapons-and-operational-risk
U.S. Air Force. (2024) Air Force Doctrine Publication 3-60: Targeting. Curtis E. LeMay Center for Doctrine Development and Education. Available from: https://www.doctrine.af.mil/Doctrine-Publications/AFDP-3-60-Targeting/
Work, R. O., & Brimley, S. (2014) 20YY: Preparing for war in the robotic age. Center for a New American Security. Available from: https://www.cnas.org/publications/reports/20yy-preparing-for-war-in-the-robotic-age